Openai project, which is engaged in the development of publicly available projects in the field of artificial intelligence, published Activations related to the speech recognition system whisper . Including the code of the reference implementation on the basis of the Pytorch framework and the set of already trained models ready for use. The code is open under the MIT license. It is claimed that for speech in English, the system provides levels of reliability and accuracy of automatic recognition close to human recognition.
For the training of the model, 680 thousand hours of speech data are used, collected from various collections covering different languages and thematic areas. About 1/3 involved in learning speech data falls into languages that are different from English. The proposed system, including correctly processes situations such as pronunciation with an accent, the presence of background noise and the use of technical jargon. In addition to speech transcription into text, the system can also translate speech from an arbitrary language into English and determine the appearance of speech in the sound flow.
models are formed in two performances: a module for English and a multilingual model, which maintains also Russian, Ukrainian and Belarusian languages. In turn, each representation is divided into 5 options that differ in size and the number of parameters covered in the model. The larger the size, the greater the accuracy and quality of recognition, but also the higher requirements for the size of the GPU video memory and lower performance. For example, the minimum option includes 39 million parameters and requires 1 GB of video memory, and the maximum includes 1550 million parameters and requires 10 GB of video memory. The minimum option is 32 times faster than the maximum.
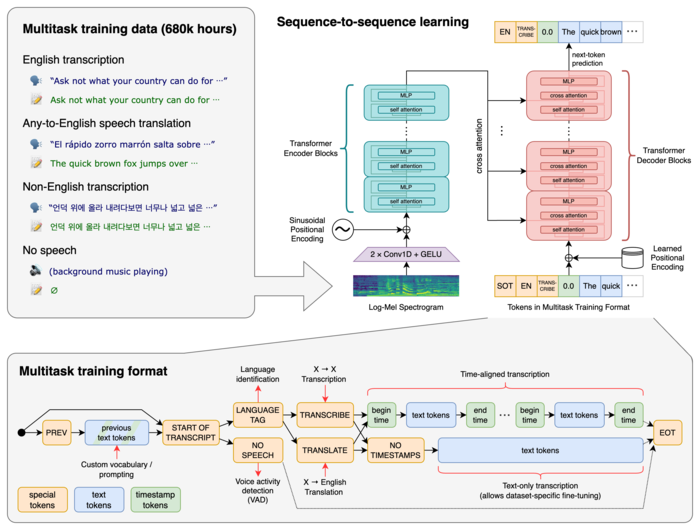
In the system used architecture of the neural network “ Transformer “, Including the encoder and decoder. The sound is divided into 30-second excerpts, which are converted into a LOG-Mel spectral and transmitted to the encoder. The result of the encoder’s work is sent to the decoder, which predicts a textual representation mixed with special tokens that allow in one common model to solve such problems as determining the language, separation of phrases by time, transcription of speech in different languages and translation into English.