A group of researchers from Tel Aviv University presented HyperStyle , inverted version developed by NVIDIA StyleGan2 machine learning system, which has been recycled to recreate missing parts when editing real images. The code is written in Python using a Pytorch framework and spreads under the MIT license.
If Stylegan allows you to synthesize realistic people looking new people, setting parameters such as age, floor, hair length, smile character, nose, skin color, leather glasses, glasses and angles, then hyperstyle makes it possible to change similar parameters in already existing photos without changing their characteristic features and maintaining the recognition of the original person. For example, with HyperStyle, you can simulate a change in the age of a person in the photo, change the hairstyle, add glasses, beard or mustache, give the image a view of a cartoon character or hand drawn pattern, making facial expression sad or cheerful. At the same time, the system can be trained not only to distort people of people, but also for any items, for example, to edit car images.
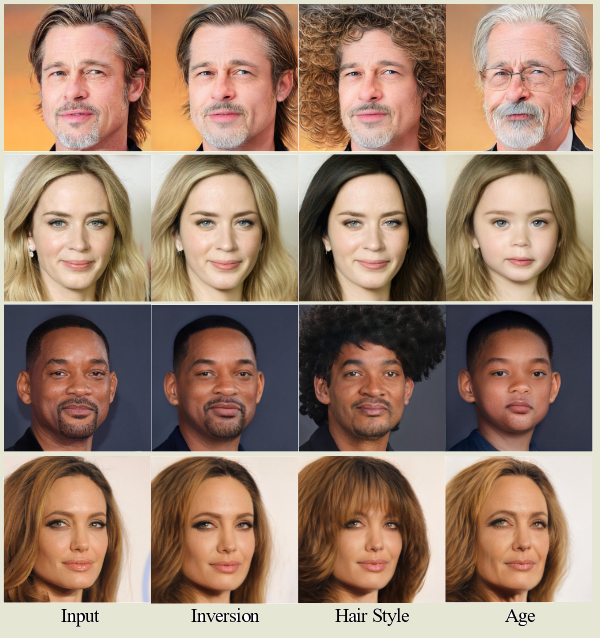
The proposed method is aimed at solving a problem with the reconstruction of the missing parts of the image when editing. In earlier, The compromise of the reconstruction and editing of the methods were proposed Methods decided through the thin setting of the image generator for substitution of the parts of the target image at the recreation of the initially absent editable regions. The disadvantage of such approaches is the need for a long-term target neural network learning for each image.
The STYLEGAN algorithm method allows you to use a typical model previously trained on common image collections, to generate the characteristic initial image elements with a level of reliability comparable to algorithms that require an individual model workout for each image. The advantages of the new method also notes the possibility of modifying images with a capacity close to the real-time mode.
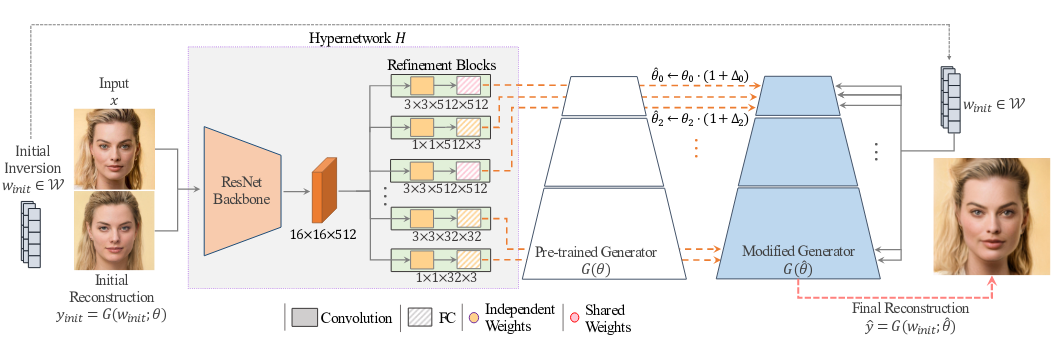
Ready-made trained models Prepared For people, machinery and animals based on collections Flickr-Faces-HQ (FFHQ, 70 thousand high-quality PNG images of people people), stanford Cars